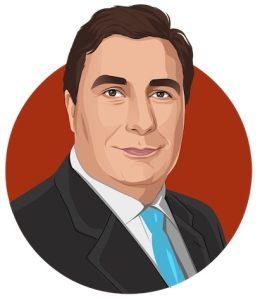
Thomas Shrager: Superior Value Outside the U.S
In a comprehensive interview, Thomas Shrager and Jay Hill from the renowned New York value investing boutique Tweedy, Browne articulate their belief that the most compelling investment opportunities currently lie outside the United States. The veteran fund managers, who oversee portfolios for the 104-year-old firm, explain that international markets offer dramatically better value propositions than the overvalued U.S. equity market, which they describe as “priced for perfection” at 24 times earnings.